學習資料
開始學習
1. SIFT特征的性質
SIFT特征不只具有尺度不變性,即使改變圖像的旋轉角度,亮度或拍攝視角,仍然能夠得到好的檢測效果。
2. SIFT算法的流程
2.1 構建尺度空間
這是一個初始化操作,尺度空間理論目的是模擬圖像數據的多尺度特征。高斯卷積核是實現尺度變換的唯一線性核,于是一副二維圖像的尺度空間定義為:

其中 G(x,y,σ) 是尺度可變高斯函數

(x,y)是空間坐標,是尺度坐標。σ大小決定圖像的平滑程度,大尺度對應圖像的概貌特征,小尺度對應圖像的細節特征。大的σ值對應粗糙尺度(低分辨率),反之,對應精細尺度(高分辨率)。為了有效的在尺度空間檢測到穩定的關鍵點,提出了高斯差分尺度空間(DOG scale-space)。利用不同尺度的高斯差分核與圖像卷積生成。

下圖所示不同σ下圖像尺度空間:
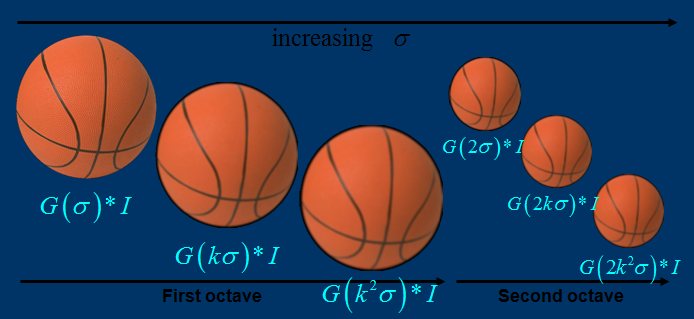
關于尺度空間的理解說明:2kσ中的2是必須的,尺度空間是連續的。在 Lowe的論文中 ,將第0層的初始尺度定為1.6(最模糊),圖片的初始尺度定為0.5(最清晰). 在檢測極值點前對原始圖像的高斯平滑以致圖像丟失高頻信息,所以 Lowe 建議在建立尺度空間前首先對原始圖像長寬擴展一倍,以保留原始圖像信息,增加特征點數量。尺度越大圖像越模糊。
圖像金字塔的建立:對于一幅圖像I,建立其在不同尺度(scale)的圖像,也成為子八度(octave),這是為了scale-invariant,也就是在任何尺度都能夠有對應的特征點,第一個子八度的scale為原圖大小,后面每個octave為上一個octave降采樣的結果,即原圖的1/4(長寬分別減半),構成下一個子八度(高一層金字塔)。

尺度空間的所有取值,i為octave的塔數(第幾個塔),s為每塔層數
由圖片size決定建幾個塔,每塔幾(S)層圖像(S一般為3-5層)。0塔的第0層是原始圖像(或你double后的圖像),往上每一層是對其下一層進行Laplacian變換(高斯卷積,其中σ值漸大,例如可以是σ, kσ, kk*σ…),直觀上看來越往上圖片越模糊。塔間的圖片是降采樣關系,例如1塔的第0層可以由0塔的第3層down sample得到,然后進行與0塔類似的高斯卷積操作。
2.2 LoG近似DoG找到關鍵點(檢測DOG尺度空間極值點)
注意:使用Difference of Gaussian圖像的極大極小值近似尋找特征點計算簡單,是尺度歸一化的LoG算子的近似
為了尋找尺度空間的極值點,每一個采樣點要和它所有的相鄰點比較,看其是否比它的圖像域和尺度域的相鄰點大或者小。
一個點如果在DOG尺度空間本層以及上下兩層的26個領域中是最大或最小值時,就認為該點是圖像在該尺度下的一個特征點,如圖所示。
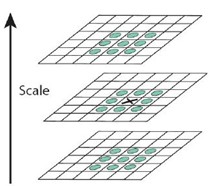
整個高斯金字塔如下圖所示,其中每個Octave代表一個金字塔,同一個金字塔內圖像尺寸一樣,同一個金字塔內每張圖通過不同的高斯卷積核產生。
在極值比較的過程中,每一組圖像的首末兩層是無法進行極值比較的,為了滿足尺度變化的連續性,我們在每一組圖像的頂層繼續用高斯模糊生成了 3 幅圖像,高斯金字塔有每組S+3層圖像。DOG金字塔每組有S+2層圖像。
解釋下什么叫“為了滿足尺度變化的連續性”:
假設s=3,也就是每個塔里有3層,則k=21/s=21/3,那么按照上圖可得Gauss Space和DoG space 分別有3個(s個)
和2個(s-1個)分量,在DoG space中,1st-octave兩項分別是σ,kσ; 2nd-octave兩項分別是2σ,2kσ;
由于無法比較極值,我們必須在高斯空間繼續添加高斯模糊項,使得形成σ,kσ,k2σ,k3σ,k4σ(?)這樣就可以選擇
DoG space中的中間三項kσ,k2σ,k3σ(只有左右都有才能有極值),那么下一octave中(由上一層降采樣獲得)
所得三項即為2kσ,2k2σ,2k3σ,其首項2kσ=24/3。剛好與上一octave末項k3σ=23/3尺度變化連續起來,所以每次要在
Gaussian space添加3項,每組(塔)共S+3層圖像,相應的DoG金字塔有S+2層圖像。
使用Laplacian of Gaussian能夠很好地找到找到圖像中的興趣點,但是需要大量的計算量,所以使用Difference of Gaussian圖像的極大極小值近似尋找特征點.DOG算子計算簡單,是尺度歸一化的LoG算子的近似,有關DOG尋找特征點的介紹及方法詳見http://blog.csdn.net/abcjennifer/article/details/7639488,極值點檢測用的Non-Maximal Suppression。
3. 除去不好的特征點
這一步本質上要去掉DoG局部曲率非常不對稱的像素。(不理解)
通過擬和三維二次函數以精確確定關鍵點的位置
和尺度
(達到亞像素精度),同時去除低對比度的關鍵點和不穩定的邊緣響應點(因為DoG算子會產生較強的邊緣響應),以增強匹配穩定性、提高抗噪聲能力,在這里使用近似Harris Corner檢測器。
計算過程摘錄如下:(還沒有自行推導)
①空間尺度函數泰勒展開式如下:

對上式求導,并令其為0,得到精確的位置, 得
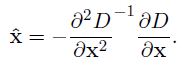
②在已經檢測到的特征點中,要去掉低對比度的特征點和不穩定的邊緣響應點。去除低對比度的點:把公式(2)代入公式(1),即在DoG Space的極值點處D(x)取值,只取前兩項可得:

若

該特征點就保留下來,否則丟棄。
③邊緣響應的去除一個定義不好的高斯差分算子的極值在橫跨邊緣的地方有較大的主曲率,而在垂直邊緣的方向有較小的主曲率。主曲率通過一個2×2 的Hessian矩陣H求出:

導數由采樣點相鄰差估計得到。
D的主曲率和H的特征值成正比,令α為較大特征值,β為較小的特征值,則

令α=γβ,則

的值在兩個特征值相等的時候最小,隨著r的增大而增大,因此,為了檢測主曲率是否在某域值r下,只需檢測

if (α+β)/ αβ> (r+1)2
/r, throw it out. 在Lowe的文章中,取r=10。
4. 給特征點賦值一個128維方向參數
上一步中確定了每幅圖中的特征點,為每個特征點計算一個方向,依照這個方向做進一步的計算, 利用關鍵點鄰域像素的梯度方向分布特性為每個關鍵點指定方向參數,使算子具備旋轉不變性。
為(x,y)處梯度的模值和方向公式。
其中L所用的尺度為每個關鍵點各自所在的尺度。至此,
圖像的關鍵點檢測完畢
,每個關鍵點有三個信息:位置
,所處尺度
、方向
,由此可以確定一個SIFT特征區域。
個人理解上面求特征點梯度方向和賦值的算式,本質上是求特征點水平和垂直方向像素值構成的兩個向量的夾角和距離。
梯度直方圖的范圍是0~360度,其中每10度一個柱,總共36個柱。隨著距 中心點越遠的領域其對直方圖的貢獻也響應減小。Lowe論文中還提到要使用高斯函數對直方圖進行平滑,減少突變的影響。這主要是因為SIFT算法只考慮了尺度和旋轉不變形,沒有考慮仿射不變性。通過高斯平滑,可以使關鍵點附近的梯度幅值有較大權重,從而部分彌補沒考慮仿射不變形產生的特征點不穩定。
通常離散的梯度直方圖要進行插值擬合處理,以求取更精確的方向角度值。
在實際計算時,我們在以關鍵點為中心的鄰域窗口內采樣,并用直方圖統計鄰域像素的梯度方向。梯度直方圖的范圍是0~360度,其中每45度一個柱,總共8個柱, 或者每10度一個柱,總共36個柱。Lowe論文中還提到要使用高斯函數對直方圖進行平滑,減少突變的影響。直方圖的峰值則代表了該關鍵點處鄰域梯度的主方向,即作為該關鍵點的方向。
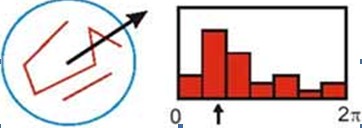
直方圖中的峰值就是主方向,其他的達到最大值80%的方向可作為輔助方向

由梯度方向直方圖確定主梯度方向
該步中將建立所有scale中特征點的描述子(128維)
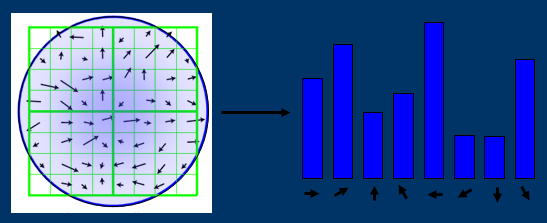
Identify peak and assign orientation and sum of magnitude to key point.
** The user may choose a threshold to exclude key points based on their** assigned sum of magnitudes.
直方圖峰值代表該關鍵點處鄰域內圖像梯度的主方向,也就是該關鍵點的主方向。在梯度方向直方圖中,當存在另一個相當于主峰值 80%能量的峰值時,則將這個方向認為是該關鍵點的輔方向。所以一個關鍵點可能檢測得到多個方向,這可以增強匹配的魯棒性。Lowe的論文指出大概有15%關鍵點具有多方向,但這些點對匹配的穩定性至為關鍵。
獲得圖像關鍵點主方向后,每個關鍵點有三個信息(x,y,σ,θ):位置、尺度、方向。由此我們可以確定一個SIFT特征區域。通常使用一個帶箭頭的圓或直接使用箭頭表示SIFT區域的三個值:中心表示特征點位置,半徑表示關鍵點尺度(r=2.5σ),箭頭表示主方向。具有多個方向的關鍵點可以復制成多份,然后將方向值分別賦給復制后的關鍵點。如下圖:
關鍵點描述子的生成步驟
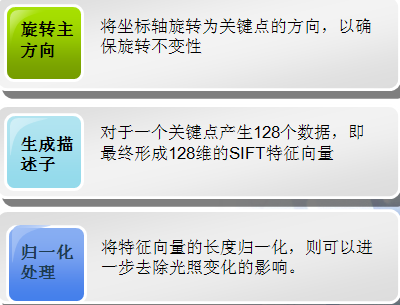
通過對關鍵點周圍圖像區域分塊,計算塊內梯度直方圖,生成具有獨特性的向量,這個向量是該區域圖像信息的一種抽象,具有唯一性。
5.關鍵點描述子的生成
首先將坐標軸旋轉為關鍵點的方向,以確保旋轉不變性。以關鍵點為中心取8×8的窗口。
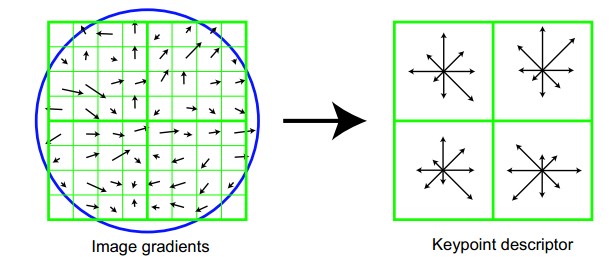
Figure.16*16的圖中其中1/4的特征點梯度方向及scale,右圖為其加權到8個主方向后的效果。
圖左部分的中央為當前關鍵點的位置,每個小格代表關鍵點鄰域所在尺度空間的一個像素,利用公式求得每個像素的梯度幅值與梯度方向,箭頭方向代表該像素的梯度方向,箭頭長度代表梯度模值,然后用高斯窗口對其進行加權運算。
圖中藍色的圈代表高斯加權的范圍(越靠近關鍵點的像素梯度方向信息貢獻越大)。然后在每4×4的小塊上計算8個方向的梯度方向直方圖,繪制每個梯度方向的累加值,即可形成一個種子點,如圖右部分示。此圖中一個關鍵點由2×2共4個種子點組成,每個種子點有8個方向向量信息。這種鄰域方向性信息聯合的思想增強了算法抗噪聲的能力,同時對于含有定位誤差的特征匹配也提供了較好的容錯性。
計算keypoint周圍的16*16的window中每一個像素的梯度,而且使用高斯下降函數降低遠離中心的權重。
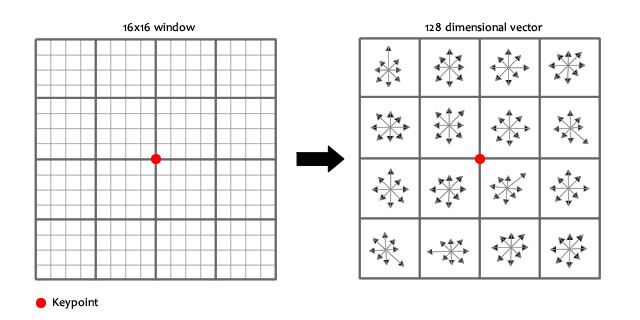
在每個44的1/16象限中,通過加權梯度值加到直方圖8個方向區間中的一個,計算出一個梯度方向直方圖。
這樣就可以對每個feature形成一個448=128維的描述子,每一維都可以表示44個格子中一個的scale/orientation. 將這個向量歸一化之后,就進一步去除了光照的影響。
6. 根據SIFT進行Match
生成了A、B兩幅圖的描述子,(分別是k1128維和k2128維),就將兩圖中各個scale(所有scale)的描述子進行匹配,匹配上128維即可表示兩個特征點match上了。
實際計算過程中,為了增強匹配的穩健性,Lowe建議對每個關鍵點使用4×4共16個種子點來描述,這樣對于一個關鍵點就可以產生128個數據,即最終形成128維的SIFT特征向量。此時SIFT特征向量已經去除了尺度變化、旋轉等幾何變形因素的影響,再繼續將特征向量的長度歸一化,則可以進一步去除光照變化的影響。 當兩幅圖像的SIFT特征向量生成后,下一步我們采用關鍵點特征向量的歐式距離來作為兩幅圖像中關鍵點的相似性判定度量。取圖像1中的某個關鍵點,并找出其與圖像2中歐式距離最近的前兩個關鍵點,在這兩個關鍵點中,如果最近的距離除以次近的距離少于某個比例閾值,則接受這一對匹配點。降低這個比例閾值,SIFT匹配點數目會減少,但更加穩定。為了排除因為圖像遮擋和背景混亂而產生的無匹配關系的關鍵點,Lowe提出了比較最近鄰距離與次近鄰距離的方法,距離比率ratio小于某個閾值的認為是正確匹配。因為對于錯誤匹配,由于特征空間的高維性,相似的距離可能有大量其他的錯誤匹配,從而它的ratio值比較高。Lowe推薦ratio的閾值為0.8。但作者對大量任意存在尺度、旋轉和亮度變化的兩幅圖片進行匹配,結果表明ratio取值在0. 4~0. 6之間最佳,小于0. 4的很少有匹配點,大于0. 6的則存在大量錯誤匹配點。(如果這個地方你要改進,最好給出一個匹配率和ration之間的關系圖,這樣才有說服力)作者建議ratio的取值原則如下:
ratio=0. 4 對于準確度要求高的匹配;ratio=0. 6 對于匹配點數目要求比較多的匹配; ratio=0. 5 一般情況下。也可按如下原則:當最近鄰距離<200時ratio=0. 6,反之ratio=0. 4。ratio的取值策略能排分錯誤匹配點。
當兩幅圖像的SIFT特征向量生成后,下一步我們采用關鍵點特征向量的歐式距離來作為兩幅圖像中關鍵點的相似性判定度量。取圖像1中的某個關鍵點,并找出其與圖像2中歐式距離最近的前兩個關鍵點,在這兩個關鍵點中,如果最近的距離除以次近的距離少于某個比例閾值,則接受這一對匹配點。降低這個比例閾值,SIFT匹配點數目會減少,但更加穩定。
7.OpenCV3.2.0版本中SIFT源碼
/*M///////////////////////////////////////////////////////////////////////////////////////
//
// IMPORTANT: READ BEFORE DOWNLOADING, COPYING, INSTALLING OR USING.
//
// By downloading, copying, installing or using the software you agree to this license.
// If you do not agree to this license, do not download, install,
// copy or use the software.
//
//
// License Agreement
// For Open Source Computer Vision Library
//
// Copyright (C) 2000-2008, Intel Corporation, all rights reserved.
// Copyright (C) 2009, Willow Garage Inc., all rights reserved.
// Third party copyrights are property of their respective owners.
//
// Redistribution and use in source and binary forms, with or without modification,
// are permitted provided that the following conditions are met:
//
// * Redistribution's of source code must retain the above copyright notice,
// this list of conditions and the following disclaimer.
//
// * Redistribution's in binary form must reproduce the above copyright notice,
// this list of conditions and the following disclaimer in the documentation
// and/or other materials provided with the distribution.
//
// * The name of the copyright holders may not be used to endorse or promote products
// derived from this software without specific prior written permission.
//
// This software is provided by the copyright holders and contributors "as is" and
// any express or implied warranties, including, but not limited to, the implied
// warranties of merchantability and fitness for a particular purpose are disclaimed.
// In no event shall the Intel Corporation or contributors be liable for any direct,
// indirect, incidental, special, exemplary, or consequential damages
// (including, but not limited to, procurement of substitute goods or services;
// loss of use, data, or profits; or business interruption) however caused
// and on any theory of liability, whether in contract, strict liability,
// or tort (including negligence or otherwise) arising in any way out of
// the use of this software, even if advised of the possibility of such damage.
//
//M*/
/**********************************************************************************************\
Implementation of SIFT is based on the code from http://blogs.oregonstate.edu/hess/code/sift/
Below is the original copyright.
// Copyright (c) 2006-2010, Rob Hess <hess@eecs.oregonstate.edu>
// All rights reserved.
// The following patent has been issued for methods embodied in this
// software: "Method and apparatus for identifying scale invariant features
// in an image and use of same for locating an object in an image," David
// G. Lowe, US Patent 6,711,293 (March 23, 2004). Provisional application
// filed March 8, 1999. Asignee: The University of British Columbia. For
// further details, contact David Lowe (lowe@cs.ubc.ca) or the
// University-Industry Liaison Office of the University of British
// Columbia.
// Note that restrictions imposed by this patent (and possibly others)
// exist independently of and may be in conflict with the freedoms granted
// in this license, which refers to copyright of the program, not patents
// for any methods that it implements. Both copyright and patent law must
// be obeyed to legally use and redistribute this program and it is not the
// purpose of this license to induce you to infringe any patents or other
// property right claims or to contest validity of any such claims. If you
// redistribute or use the program, then this license merely protects you
// from committing copyright infringement. It does not protect you from
// committing patent infringement. So, before you do anything with this
// program, make sure that you have permission to do so not merely in terms
// of copyright, but also in terms of patent law.
// Please note that this license is not to be understood as a guarantee
// either. If you use the program according to this license, but in
// conflict with patent law, it does not mean that the licensor will refund
// you for any losses that you incur if you are sued for your patent
// infringement.
// Redistribution and use in source and binary forms, with or without
// modification, are permitted provided that the following conditions are
// met:
// * Redistributions of source code must retain the above copyright and
// patent notices, this list of conditions and the following
// disclaimer.
// * Redistributions in binary form must reproduce the above copyright
// notice, this list of conditions and the following disclaimer in
// the documentation and/or other materials provided with the
// distribution.
// * Neither the name of Oregon State University nor the names of its
// contributors may be used to endorse or promote products derived
// from this software without specific prior written permission.
// THIS SOFTWARE IS PROVIDED BY THE COPYRIGHT HOLDERS AND CONTRIBUTORS "AS
// IS" AND ANY EXPRESS OR IMPLIED WARRANTIES, INCLUDING, BUT NOT LIMITED
// TO, THE IMPLIED WARRANTIES OF MERCHANTABILITY AND FITNESS FOR A
// PARTICULAR PURPOSE ARE DISCLAIMED. IN NO EVENT SHALL THE COPYRIGHT
// HOLDER BE LIABLE FOR ANY DIRECT, INDIRECT, INCIDENTAL, SPECIAL,
// EXEMPLARY, OR CONSEQUENTIAL DAMAGES (INCLUDING, BUT NOT LIMITED TO,
// PROCUREMENT OF SUBSTITUTE GOODS OR SERVICES; LOSS OF USE, DATA, OR
// PROFITS; OR BUSINESS INTERRUPTION) HOWEVER CAUSED AND ON ANY THEORY OF
// LIABILITY, WHETHER IN CONTRACT, STRICT LIABILITY, OR TORT (INCLUDING
// NEGLIGENCE OR OTHERWISE) ARISING IN ANY WAY OUT OF THE USE OF THIS
// SOFTWARE, EVEN IF ADVISED OF THE POSSIBILITY OF SUCH DAMAGE.
\**********************************************************************************************/
#include "precomp.hpp"
#include <iostream>
#include <stdarg.h>
#include <opencv2/core/hal/hal.hpp>
namespace cv
{
namespace xfeatures2d
{
/*!
SIFT implementation.
The class implements SIFT algorithm by D. Lowe.
*/
class SIFT_Impl : public SIFT
{
public:
explicit SIFT_Impl( int nfeatures = 0, int nOctaveLayers = 3,
double contrastThreshold = 0.04, double edgeThreshold = 10,
double sigma = 1.6);
//! returns the descriptor size in floats (128)
int descriptorSize() const;
//! returns the descriptor type
int descriptorType() const;
//! returns the default norm type
int defaultNorm() const;
//! finds the keypoints and computes descriptors for them using SIFT algorithm.
//! Optionally it can compute descriptors for the user-provided keypoints
void detectAndCompute(InputArray img, InputArray mask,
std::vector<KeyPoint>& keypoints,
OutputArray descriptors,
bool useProvidedKeypoints = false);
void buildGaussianPyramid( const Mat& base, std::vector<Mat>& pyr, int nOctaves ) const;
void buildDoGPyramid( const std::vector<Mat>& pyr, std::vector<Mat>& dogpyr ) const;
void findScaleSpaceExtrema( const std::vector<Mat>& gauss_pyr, const std::vector<Mat>& dog_pyr,
std::vector<KeyPoint>& keypoints ) const;
protected:
CV_PROP_RW int nfeatures;
CV_PROP_RW int nOctaveLayers;
CV_PROP_RW double contrastThreshold;
CV_PROP_RW double edgeThreshold;
CV_PROP_RW double sigma;
};
Ptr<SIFT> SIFT::create( int _nfeatures, int _nOctaveLayers,
double _contrastThreshold, double _edgeThreshold, double _sigma )
{
return makePtr<SIFT_Impl>(_nfeatures, _nOctaveLayers, _contrastThreshold, _edgeThreshold, _sigma);
}
/******************************* Defs and macros *****************************/
// default width of descriptor histogram array
static const int SIFT_DESCR_WIDTH = 4;
// default number of bins per histogram in descriptor array
static const int SIFT_DESCR_HIST_BINS = 8;
// assumed gaussian blur for input image
static const float SIFT_INIT_SIGMA = 0.5f;
// width of border in which to ignore keypoints
static const int SIFT_IMG_BORDER = 5;
// maximum steps of keypoint interpolation before failure
static const int SIFT_MAX_INTERP_STEPS = 5;
// default number of bins in histogram for orientation assignment
static const int SIFT_ORI_HIST_BINS = 36;
// determines gaussian sigma for orientation assignment
static const float SIFT_ORI_SIG_FCTR = 1.5f;
// determines the radius of the region used in orientation assignment
static const float SIFT_ORI_RADIUS = 3 * SIFT_ORI_SIG_FCTR;
// orientation magnitude relative to max that results in new feature
static const float SIFT_ORI_PEAK_RATIO = 0.8f;
// determines the size of a single descriptor orientation histogram
static const float SIFT_DESCR_SCL_FCTR = 3.f;
// threshold on magnitude of elements of descriptor vector
static const float SIFT_DESCR_MAG_THR = 0.2f;
// factor used to convert floating-point descriptor to unsigned char
static const float SIFT_INT_DESCR_FCTR = 512.f;
#if 0
// intermediate type used for DoG pyramids
typedef short sift_wt;
static const int SIFT_FIXPT_SCALE = 48;
#else
// intermediate type used for DoG pyramids
typedef float sift_wt;
static const int SIFT_FIXPT_SCALE = 1;
#endif
static inline void
unpackOctave(const KeyPoint& kpt, int& octave, int& layer, float& scale)
{
octave = kpt.octave & 255;
layer = (kpt.octave >> 8) & 255;
octave = octave < 128 ? octave : (-128 | octave);
scale = octave >= 0 ? 1.f/(1 << octave) : (float)(1 << -octave);
}
static Mat createInitialImage( const Mat& img, bool doubleImageSize, float sigma )
{
Mat gray, gray_fpt;
if( img.channels() == 3 || img.channels() == 4 )
{
cvtColor(img, gray, COLOR_BGR2GRAY);
gray.convertTo(gray_fpt, DataType<sift_wt>::type, SIFT_FIXPT_SCALE, 0);
}
else
img.convertTo(gray_fpt, DataType<sift_wt>::type, SIFT_FIXPT_SCALE, 0);
float sig_diff;
if( doubleImageSize )
{
sig_diff = sqrtf( std::max(sigma * sigma - SIFT_INIT_SIGMA * SIFT_INIT_SIGMA * 4, 0.01f) );
Mat dbl;
resize(gray_fpt, dbl, Size(gray_fpt.cols*2, gray_fpt.rows*2), 0, 0, INTER_LINEAR);
GaussianBlur(dbl, dbl, Size(), sig_diff, sig_diff);
return dbl;
}
else
{
sig_diff = sqrtf( std::max(sigma * sigma - SIFT_INIT_SIGMA * SIFT_INIT_SIGMA, 0.01f) );
GaussianBlur(gray_fpt, gray_fpt, Size(), sig_diff, sig_diff);
return gray_fpt;
}
}
void SIFT_Impl::buildGaussianPyramid( const Mat& base, std::vector<Mat>& pyr, int nOctaves ) const
{
std::vector<double> sig(nOctaveLayers + 3);
pyr.resize(nOctaves*(nOctaveLayers + 3));
// precompute Gaussian sigmas using the following formula:
// \sigma_{total}^2 = \sigma_{i}^2 + \sigma_{i-1}^2
sig[0] = sigma;
double k = std::pow( 2., 1. / nOctaveLayers );
for( int i = 1; i < nOctaveLayers + 3; i++ )
{
double sig_prev = std::pow(k, (double)(i-1))*sigma;
double sig_total = sig_prev*k;
sig[i] = std::sqrt(sig_total*sig_total - sig_prev*sig_prev);
}
for( int o = 0; o < nOctaves; o++ )
{
for( int i = 0; i < nOctaveLayers + 3; i++ )
{
Mat& dst = pyr[o*(nOctaveLayers + 3) + i];
if( o == 0 && i == 0 )
dst = base;
// base of new octave is halved image from end of previous octave
else if( i == 0 )
{
const Mat& src = pyr[(o-1)*(nOctaveLayers + 3) + nOctaveLayers];
resize(src, dst, Size(src.cols/2, src.rows/2),
0, 0, INTER_NEAREST);
}
else
{
const Mat& src = pyr[o*(nOctaveLayers + 3) + i-1];
GaussianBlur(src, dst, Size(), sig[i], sig[i]);
}
}
}
}
void SIFT_Impl::buildDoGPyramid( const std::vector<Mat>& gpyr, std::vector<Mat>& dogpyr ) const
{
int nOctaves = (int)gpyr.size()/(nOctaveLayers + 3);
dogpyr.resize( nOctaves*(nOctaveLayers + 2) );
for( int o = 0; o < nOctaves; o++ )
{
for( int i = 0; i < nOctaveLayers + 2; i++ )
{
const Mat& src1 = gpyr[o*(nOctaveLayers + 3) + i];
const Mat& src2 = gpyr[o*(nOctaveLayers + 3) + i + 1];
Mat& dst = dogpyr[o*(nOctaveLayers + 2) + i];
subtract(src2, src1, dst, noArray(), DataType<sift_wt>::type);
}
}
}
// Computes a gradient orientation histogram at a specified pixel
static float calcOrientationHist( const Mat& img, Point pt, int radius,
float sigma, float* hist, int n )
{
int i, j, k, len = (radius*2+1)*(radius*2+1);
float expf_scale = -1.f/(2.f * sigma * sigma);
AutoBuffer<float> buf(len*4 + n+4);
float *X = buf, *Y = X + len, *Mag = X, *Ori = Y + len, *W = Ori + len;
float* temphist = W + len + 2;
for( i = 0; i < n; i++ )
temphist[i] = 0.f;
for( i = -radius, k = 0; i <= radius; i++ )
{
int y = pt.y + i;
if( y <= 0 || y >= img.rows - 1 )
continue;
for( j = -radius; j <= radius; j++ )
{
int x = pt.x + j;
if( x <= 0 || x >= img.cols - 1 )
continue;
float dx = (float)(img.at<sift_wt>(y, x+1) - img.at<sift_wt>(y, x-1));
float dy = (float)(img.at<sift_wt>(y-1, x) - img.at<sift_wt>(y+1, x));
X[k] = dx; Y[k] = dy; W[k] = (i*i + j*j)*expf_scale;
k++;
}
}
len = k;
// compute gradient values, orientations and the weights over the pixel neighborhood
cv::hal::exp32f(W, W, len);
cv::hal::fastAtan2(Y, X, Ori, len, true);
cv::hal::magnitude32f(X, Y, Mag, len);
for( k = 0; k < len; k++ )
{
int bin = cvRound((n/360.f)*Ori[k]);
if( bin >= n )
bin -= n;
if( bin < 0 )
bin += n;
temphist[bin] += W[k]*Mag[k];
}
// smooth the histogram
temphist[-1] = temphist[n-1];
temphist[-2] = temphist[n-2];
temphist[n] = temphist[0];
temphist[n+1] = temphist[1];
for( i = 0; i < n; i++ )
{
hist[i] = (temphist[i-2] + temphist[i+2])*(1.f/16.f) +
(temphist[i-1] + temphist[i+1])*(4.f/16.f) +
temphist[i]*(6.f/16.f);
}
float maxval = hist[0];
for( i = 1; i < n; i++ )
maxval = std::max(maxval, hist[i]);
return maxval;
}
//
// Interpolates a scale-space extremum's location and scale to subpixel
// accuracy to form an image feature. Rejects features with low contrast.
// Based on Section 4 of Lowe's paper.
static bool adjustLocalExtrema( const std::vector<Mat>& dog_pyr, KeyPoint& kpt, int octv,
int& layer, int& r, int& c, int nOctaveLayers,
float contrastThreshold, float edgeThreshold, float sigma )
{
const float img_scale = 1.f/(255*SIFT_FIXPT_SCALE);
const float deriv_scale = img_scale*0.5f;
const float second_deriv_scale = img_scale;
const float cross_deriv_scale = img_scale*0.25f;
float xi=0, xr=0, xc=0, contr=0;
int i = 0;
for( ; i < SIFT_MAX_INTERP_STEPS; i++ )
{
int idx = octv*(nOctaveLayers+2) + layer;
const Mat& img = dog_pyr[idx];
const Mat& prev = dog_pyr[idx-1];
const Mat& next = dog_pyr[idx+1];
Vec3f dD((img.at<sift_wt>(r, c+1) - img.at<sift_wt>(r, c-1))*deriv_scale,
(img.at<sift_wt>(r+1, c) - img.at<sift_wt>(r-1, c))*deriv_scale,
(next.at<sift_wt>(r, c) - prev.at<sift_wt>(r, c))*deriv_scale);
float v2 = (float)img.at<sift_wt>(r, c)*2;
float dxx = (img.at<sift_wt>(r, c+1) + img.at<sift_wt>(r, c-1) - v2)*second_deriv_scale;
float dyy = (img.at<sift_wt>(r+1, c) + img.at<sift_wt>(r-1, c) - v2)*second_deriv_scale;
float dss = (next.at<sift_wt>(r, c) + prev.at<sift_wt>(r, c) - v2)*second_deriv_scale;
float dxy = (img.at<sift_wt>(r+1, c+1) - img.at<sift_wt>(r+1, c-1) -
img.at<sift_wt>(r-1, c+1) + img.at<sift_wt>(r-1, c-1))*cross_deriv_scale;
float dxs = (next.at<sift_wt>(r, c+1) - next.at<sift_wt>(r, c-1) -
prev.at<sift_wt>(r, c+1) + prev.at<sift_wt>(r, c-1))*cross_deriv_scale;
float dys = (next.at<sift_wt>(r+1, c) - next.at<sift_wt>(r-1, c) -
prev.at<sift_wt>(r+1, c) + prev.at<sift_wt>(r-1, c))*cross_deriv_scale;
Matx33f H(dxx, dxy, dxs,
dxy, dyy, dys,
dxs, dys, dss);
Vec3f X = H.solve(dD, DECOMP_LU);
xi = -X[2];
xr = -X[1];
xc = -X[0];
if( std::abs(xi) < 0.5f && std::abs(xr) < 0.5f && std::abs(xc) < 0.5f )
break;
if( std::abs(xi) > (float)(INT_MAX/3) ||
std::abs(xr) > (float)(INT_MAX/3) ||
std::abs(xc) > (float)(INT_MAX/3) )
return false;
c += cvRound(xc);
r += cvRound(xr);
layer += cvRound(xi);
if( layer < 1 || layer > nOctaveLayers ||
c < SIFT_IMG_BORDER || c >= img.cols - SIFT_IMG_BORDER ||
r < SIFT_IMG_BORDER || r >= img.rows - SIFT_IMG_BORDER )
return false;
}
// ensure convergence of interpolation
if( i >= SIFT_MAX_INTERP_STEPS )
return false;
{
int idx = octv*(nOctaveLayers+2) + layer;
const Mat& img = dog_pyr[idx];
const Mat& prev = dog_pyr[idx-1];
const Mat& next = dog_pyr[idx+1];
Matx31f dD((img.at<sift_wt>(r, c+1) - img.at<sift_wt>(r, c-1))*deriv_scale,
(img.at<sift_wt>(r+1, c) - img.at<sift_wt>(r-1, c))*deriv_scale,
(next.at<sift_wt>(r, c) - prev.at<sift_wt>(r, c))*deriv_scale);
float t = dD.dot(Matx31f(xc, xr, xi));
contr = img.at<sift_wt>(r, c)*img_scale + t * 0.5f;
if( std::abs( contr ) * nOctaveLayers < contrastThreshold )
return false;
// principal curvatures are computed using the trace and det of Hessian
float v2 = img.at<sift_wt>(r, c)*2.f;
float dxx = (img.at<sift_wt>(r, c+1) + img.at<sift_wt>(r, c-1) - v2)*second_deriv_scale;
float dyy = (img.at<sift_wt>(r+1, c) + img.at<sift_wt>(r-1, c) - v2)*second_deriv_scale;
float dxy = (img.at<sift_wt>(r+1, c+1) - img.at<sift_wt>(r+1, c-1) -
img.at<sift_wt>(r-1, c+1) + img.at<sift_wt>(r-1, c-1)) * cross_deriv_scale;
float tr = dxx + dyy;
float det = dxx * dyy - dxy * dxy;
if( det <= 0 || tr*tr*edgeThreshold >= (edgeThreshold + 1)*(edgeThreshold + 1)*det )
return false;
}
kpt.pt.x = (c + xc) * (1 << octv);
kpt.pt.y = (r + xr) * (1 << octv);
kpt.octave = octv + (layer << 8) + (cvRound((xi + 0.5)*255) << 16);
kpt.size = sigma*powf(2.f, (layer + xi) / nOctaveLayers)*(1 << octv)*2;
kpt.response = std::abs(contr);
return true;
}
//
// Detects features at extrema in DoG scale space. Bad features are discarded
// based on contrast and ratio of principal curvatures.
void SIFT_Impl::findScaleSpaceExtrema( const std::vector<Mat>& gauss_pyr, const std::vector<Mat>& dog_pyr,
std::vector<KeyPoint>& keypoints ) const
{
int nOctaves = (int)gauss_pyr.size()/(nOctaveLayers + 3);
int threshold = cvFloor(0.5 * contrastThreshold / nOctaveLayers * 255 * SIFT_FIXPT_SCALE);
const int n = SIFT_ORI_HIST_BINS;
float hist[n];
KeyPoint kpt;
keypoints.clear();
for( int o = 0; o < nOctaves; o++ )
for( int i = 1; i <= nOctaveLayers; i++ )
{
int idx = o*(nOctaveLayers+2)+i;
const Mat& img = dog_pyr[idx];
const Mat& prev = dog_pyr[idx-1];
const Mat& next = dog_pyr[idx+1];
int step = (int)img.step1();
int rows = img.rows, cols = img.cols;
for( int r = SIFT_IMG_BORDER; r < rows-SIFT_IMG_BORDER; r++)
{
const sift_wt* currptr = img.ptr<sift_wt>(r);
const sift_wt* prevptr = prev.ptr<sift_wt>(r);
const sift_wt* nextptr = next.ptr<sift_wt>(r);
for( int c = SIFT_IMG_BORDER; c < cols-SIFT_IMG_BORDER; c++)
{
sift_wt val = currptr[c];
// find local extrema with pixel accuracy
if( std::abs(val) > threshold &&
((val > 0 && val >= currptr[c-1] && val >= currptr[c+1] &&
val >= currptr[c-step-1] && val >= currptr[c-step] && val >= currptr[c-step+1] &&
val >= currptr[c+step-1] && val >= currptr[c+step] && val >= currptr[c+step+1] &&
val >= nextptr[c] && val >= nextptr[c-1] && val >= nextptr[c+1] &&
val >= nextptr[c-step-1] && val >= nextptr[c-step] && val >= nextptr[c-step+1] &&
val >= nextptr[c+step-1] && val >= nextptr[c+step] && val >= nextptr[c+step+1] &&
val >= prevptr[c] && val >= prevptr[c-1] && val >= prevptr[c+1] &&
val >= prevptr[c-step-1] && val >= prevptr[c-step] && val >= prevptr[c-step+1] &&
val >= prevptr[c+step-1] && val >= prevptr[c+step] && val >= prevptr[c+step+1]) ||
(val < 0 && val <= currptr[c-1] && val <= currptr[c+1] &&
val <= currptr[c-step-1] && val <= currptr[c-step] && val <= currptr[c-step+1] &&
val <= currptr[c+step-1] && val <= currptr[c+step] && val <= currptr[c+step+1] &&
val <= nextptr[c] && val <= nextptr[c-1] && val <= nextptr[c+1] &&
val <= nextptr[c-step-1] && val <= nextptr[c-step] && val <= nextptr[c-step+1] &&
val <= nextptr[c+step-1] && val <= nextptr[c+step] && val <= nextptr[c+step+1] &&
val <= prevptr[c] && val <= prevptr[c-1] && val <= prevptr[c+1] &&
val <= prevptr[c-step-1] && val <= prevptr[c-step] && val <= prevptr[c-step+1] &&
val <= prevptr[c+step-1] && val <= prevptr[c+step] && val <= prevptr[c+step+1])))
{
int r1 = r, c1 = c, layer = i;
if( !adjustLocalExtrema(dog_pyr, kpt, o, layer, r1, c1,
nOctaveLayers, (float)contrastThreshold,
(float)edgeThreshold, (float)sigma) )
continue;
float scl_octv = kpt.size*0.5f/(1 << o);
float omax = calcOrientationHist(gauss_pyr[o*(nOctaveLayers+3) + layer],
Point(c1, r1),
cvRound(SIFT_ORI_RADIUS * scl_octv),
SIFT_ORI_SIG_FCTR * scl_octv,
hist, n);
float mag_thr = (float)(omax * SIFT_ORI_PEAK_RATIO);
for( int j = 0; j < n; j++ )
{
int l = j > 0 ? j - 1 : n - 1;
int r2 = j < n-1 ? j + 1 : 0;
if( hist[j] > hist[l] && hist[j] > hist[r2] && hist[j] >= mag_thr )
{
float bin = j + 0.5f * (hist[l]-hist[r2]) / (hist[l] - 2*hist[j] + hist[r2]);
bin = bin < 0 ? n + bin : bin >= n ? bin - n : bin;
kpt.angle = 360.f - (float)((360.f/n) * bin);
if(std::abs(kpt.angle - 360.f) < FLT_EPSILON)
kpt.angle = 0.f;
keypoints.push_back(kpt);
}
}
}
}
}
}
}
static void calcSIFTDescriptor( const Mat& img, Point2f ptf, float ori, float scl,
int d, int n, float* dst )
{
Point pt(cvRound(ptf.x), cvRound(ptf.y));
float cos_t = cosf(ori*(float)(CV_PI/180));
float sin_t = sinf(ori*(float)(CV_PI/180));
float bins_per_rad = n / 360.f;
float exp_scale = -1.f/(d * d * 0.5f);
float hist_width = SIFT_DESCR_SCL_FCTR * scl;
int radius = cvRound(hist_width * 1.4142135623730951f * (d + 1) * 0.5f);
// Clip the radius to the diagonal of the image to avoid autobuffer too large exception
radius = std::min(radius, (int) sqrt(((double) img.cols)*img.cols + ((double) img.rows)*img.rows));
cos_t /= hist_width;
sin_t /= hist_width;
int i, j, k, len = (radius*2+1)*(radius*2+1), histlen = (d+2)*(d+2)*(n+2);
int rows = img.rows, cols = img.cols;
AutoBuffer<float> buf(len*6 + histlen);
float *X = buf, *Y = X + len, *Mag = Y, *Ori = Mag + len, *W = Ori + len;
float *RBin = W + len, *CBin = RBin + len, *hist = CBin + len;
for( i = 0; i < d+2; i++ )
{
for( j = 0; j < d+2; j++ )
for( k = 0; k < n+2; k++ )
hist[(i*(d+2) + j)*(n+2) + k] = 0.;
}
for( i = -radius, k = 0; i <= radius; i++ )
for( j = -radius; j <= radius; j++ )
{
// Calculate sample's histogram array coords rotated relative to ori.
// Subtract 0.5 so samples that fall e.g. in the center of row 1 (i.e.
// r_rot = 1.5) have full weight placed in row 1 after interpolation.
float c_rot = j * cos_t - i * sin_t;
float r_rot = j * sin_t + i * cos_t;
float rbin = r_rot + d/2 - 0.5f;
float cbin = c_rot + d/2 - 0.5f;
int r = pt.y + i, c = pt.x + j;
if( rbin > -1 && rbin < d && cbin > -1 && cbin < d &&
r > 0 && r < rows - 1 && c > 0 && c < cols - 1 )
{
float dx = (float)(img.at<sift_wt>(r, c+1) - img.at<sift_wt>(r, c-1));
float dy = (float)(img.at<sift_wt>(r-1, c) - img.at<sift_wt>(r+1, c));
X[k] = dx; Y[k] = dy; RBin[k] = rbin; CBin[k] = cbin;
W[k] = (c_rot * c_rot + r_rot * r_rot)*exp_scale;
k++;
}
}
len = k;
cv::hal::fastAtan2(Y, X, Ori, len, true);
cv::hal::magnitude32f(X, Y, Mag, len);
cv::hal::exp32f(W, W, len);
for( k = 0; k < len; k++ )
{
float rbin = RBin[k], cbin = CBin[k];
float obin = (Ori[k] - ori)*bins_per_rad;
float mag = Mag[k]*W[k];
int r0 = cvFloor( rbin );
int c0 = cvFloor( cbin );
int o0 = cvFloor( obin );
rbin -= r0;
cbin -= c0;
obin -= o0;
if( o0 < 0 )
o0 += n;
if( o0 >= n )
o0 -= n;
// histogram update using tri-linear interpolation
float v_r1 = mag*rbin, v_r0 = mag - v_r1;
float v_rc11 = v_r1*cbin, v_rc10 = v_r1 - v_rc11;
float v_rc01 = v_r0*cbin, v_rc00 = v_r0 - v_rc01;
float v_rco111 = v_rc11*obin, v_rco110 = v_rc11 - v_rco111;
float v_rco101 = v_rc10*obin, v_rco100 = v_rc10 - v_rco101;
float v_rco011 = v_rc01*obin, v_rco010 = v_rc01 - v_rco011;
float v_rco001 = v_rc00*obin, v_rco000 = v_rc00 - v_rco001;
int idx = ((r0+1)*(d+2) + c0+1)*(n+2) + o0;
hist[idx] += v_rco000;
hist[idx+1] += v_rco001;
hist[idx+(n+2)] += v_rco010;
hist[idx+(n+3)] += v_rco011;
hist[idx+(d+2)*(n+2)] += v_rco100;
hist[idx+(d+2)*(n+2)+1] += v_rco101;
hist[idx+(d+3)*(n+2)] += v_rco110;
hist[idx+(d+3)*(n+2)+1] += v_rco111;
}
// finalize histogram, since the orientation histograms are circular
for( i = 0; i < d; i++ )
for( j = 0; j < d; j++ )
{
int idx = ((i+1)*(d+2) + (j+1))*(n+2);
hist[idx] += hist[idx+n];
hist[idx+1] += hist[idx+n+1];
for( k = 0; k < n; k++ )
dst[(i*d + j)*n + k] = hist[idx+k];
}
// copy histogram to the descriptor,
// apply hysteresis thresholding
// and scale the result, so that it can be easily converted
// to byte array
float nrm2 = 0;
len = d*d*n;
for( k = 0; k < len; k++ )
nrm2 += dst[k]*dst[k];
float thr = std::sqrt(nrm2)*SIFT_DESCR_MAG_THR;
for( i = 0, nrm2 = 0; i < k; i++ )
{
float val = std::min(dst[i], thr);
dst[i] = val;
nrm2 += val*val;
}
nrm2 = SIFT_INT_DESCR_FCTR/std::max(std::sqrt(nrm2), FLT_EPSILON);
#if 1
for( k = 0; k < len; k++ )
{
dst[k] = saturate_cast<uchar>(dst[k]*nrm2);
}
#else
float nrm1 = 0;
for( k = 0; k < len; k++ )
{
dst[k] *= nrm2;
nrm1 += dst[k];
}
nrm1 = 1.f/std::max(nrm1, FLT_EPSILON);
for( k = 0; k < len; k++ )
{
dst[k] = std::sqrt(dst[k] * nrm1);//saturate_cast<uchar>(std::sqrt(dst[k] * nrm1)*SIFT_INT_DESCR_FCTR);
}
#endif
}
static void calcDescriptors(const std::vector<Mat>& gpyr, const std::vector<KeyPoint>& keypoints,
Mat& descriptors, int nOctaveLayers, int firstOctave )
{
int d = SIFT_DESCR_WIDTH, n = SIFT_DESCR_HIST_BINS;
for( size_t i = 0; i < keypoints.size(); i++ )
{
KeyPoint kpt = keypoints[i];
int octave, layer;
float scale;
unpackOctave(kpt, octave, layer, scale);
CV_Assert(octave >= firstOctave && layer <= nOctaveLayers+2);
float size=kpt.size*scale;
Point2f ptf(kpt.pt.x*scale, kpt.pt.y*scale);
const Mat& img = gpyr[(octave - firstOctave)*(nOctaveLayers + 3) + layer];
float angle = 360.f - kpt.angle;
if(std::abs(angle - 360.f) < FLT_EPSILON)
angle = 0.f;
calcSIFTDescriptor(img, ptf, angle, size*0.5f, d, n, descriptors.ptr<float>((int)i));
}
}
//////////////////////////////////////////////////////////////////////////////////////////
SIFT_Impl::SIFT_Impl( int _nfeatures, int _nOctaveLayers,
double _contrastThreshold, double _edgeThreshold, double _sigma )
: nfeatures(_nfeatures), nOctaveLayers(_nOctaveLayers),
contrastThreshold(_contrastThreshold), edgeThreshold(_edgeThreshold), sigma(_sigma)
{
}
int SIFT_Impl::descriptorSize() const
{
return SIFT_DESCR_WIDTH*SIFT_DESCR_WIDTH*SIFT_DESCR_HIST_BINS;
}
int SIFT_Impl::descriptorType() const
{
return CV_32F;
}
int SIFT_Impl::defaultNorm() const
{
return NORM_L2;
}
void SIFT_Impl::detectAndCompute(InputArray _image, InputArray _mask,
std::vector<KeyPoint>& keypoints,
OutputArray _descriptors,
bool useProvidedKeypoints)
{
int firstOctave = -1, actualNOctaves = 0, actualNLayers = 0;
Mat image = _image.getMat(), mask = _mask.getMat();
if( image.empty() || image.depth() != CV_8U )
CV_Error( Error::StsBadArg, "image is empty or has incorrect depth (!=CV_8U)" );
if( !mask.empty() && mask.type() != CV_8UC1 )
CV_Error( Error::StsBadArg, "mask has incorrect type (!=CV_8UC1)" );
if( useProvidedKeypoints )
{
firstOctave = 0;
int maxOctave = INT_MIN;
for( size_t i = 0; i < keypoints.size(); i++ )
{
int octave, layer;
float scale;
unpackOctave(keypoints[i], octave, layer, scale);
firstOctave = std::min(firstOctave, octave);
maxOctave = std::max(maxOctave, octave);
actualNLayers = std::max(actualNLayers, layer-2);
}
firstOctave = std::min(firstOctave, 0);
CV_Assert( firstOctave >= -1 && actualNLayers <= nOctaveLayers );
actualNOctaves = maxOctave - firstOctave + 1;
}
Mat base = createInitialImage(image, firstOctave < 0, (float)sigma);
std::vector<Mat> gpyr, dogpyr;
int nOctaves = actualNOctaves > 0 ? actualNOctaves : cvRound(std::log( (double)std::min( base.cols, base.rows ) ) / std::log(2.) - 2) - firstOctave;
//double t, tf = getTickFrequency();
//t = (double)getTickCount();
buildGaussianPyramid(base, gpyr, nOctaves);
buildDoGPyramid(gpyr, dogpyr);
//t = (double)getTickCount() - t;
//printf("pyramid construction time: %g\n", t*1000./tf);
if( !useProvidedKeypoints )
{
//t = (double)getTickCount();
findScaleSpaceExtrema(gpyr, dogpyr, keypoints);
KeyPointsFilter::removeDuplicated( keypoints );
if( nfeatures > 0 )
KeyPointsFilter::retainBest(keypoints, nfeatures);
//t = (double)getTickCount() - t;
//printf("keypoint detection time: %g\n", t*1000./tf);
if( firstOctave < 0 )
for( size_t i = 0; i < keypoints.size(); i++ )
{
KeyPoint& kpt = keypoints[i];
float scale = 1.f/(float)(1 << -firstOctave);
kpt.octave = (kpt.octave & ~255) | ((kpt.octave + firstOctave) & 255);
kpt.pt *= scale;
kpt.size *= scale;
}
if( !mask.empty() )
KeyPointsFilter::runByPixelsMask( keypoints, mask );
}
else
{
// filter keypoints by mask
//KeyPointsFilter::runByPixelsMask( keypoints, mask );
}
if( _descriptors.needed() )
{
//t = (double)getTickCount();
int dsize = descriptorSize();
_descriptors.create((int)keypoints.size(), dsize, CV_32F);
Mat descriptors = _descriptors.getMat();
calcDescriptors(gpyr, keypoints, descriptors, nOctaveLayers, firstOctave);
//t = (double)getTickCount() - t;
//printf("descriptor extraction time: %g\n", t*1000./tf);
}
}
}
}